Accelerate Your ETL Workflows with Databricks Data Management
Big Data
5 MIN READ
October 3, 2024
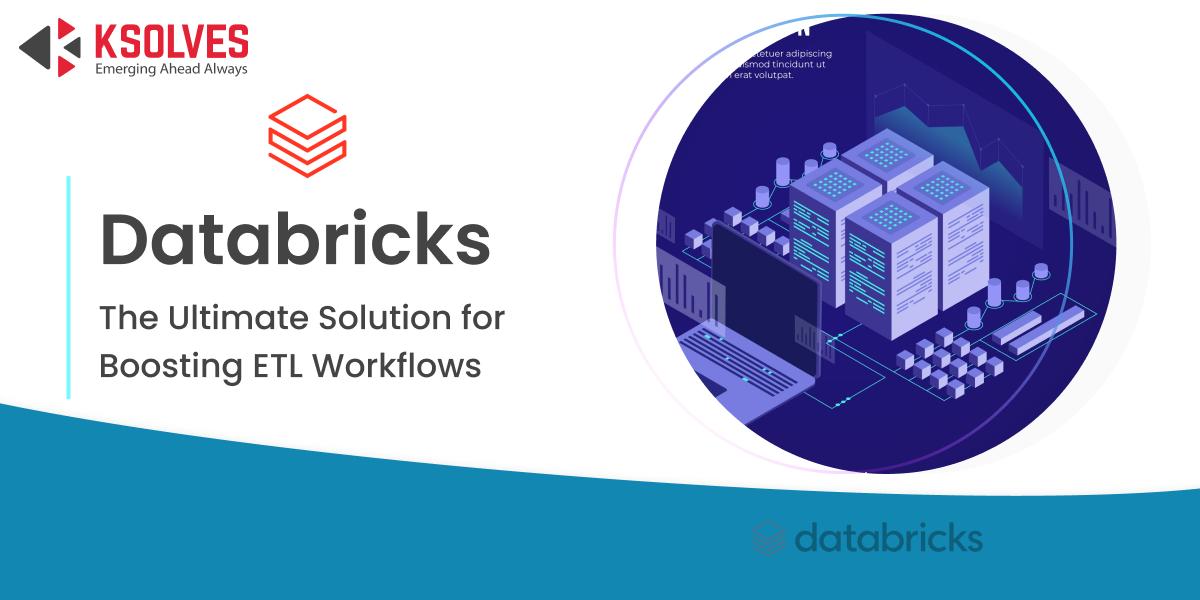
In a world overflowing with dataโfrom small businesses to large enterprisesโwe need ETL (Extract, Transform, Load) workflows to survive. Enter Databricks, the game-changing platform that takes your data management to the next level.
Databricks is changing the way businesses turn raw data into actionable insights by integrating Apache Sparkโs powerful tools and collaboration tools. Are you ready to supercharge your ETL processes? Check out why Databricks is the perfect solution for your data needs.
Explore Databricks, Your Ultimate Data Solution
Databricks is a cloud-based platform that helps companies manage large amounts of data. Itโs a powerful tool that helps businesses collect, process, and analyze data in one place. Especially in Databricks for Data Engineering. With strong features including data science and data analysis. This platform facilitates collaboration between teams. Help them work together to gain insights from your data.
How do Databricks work?
Databricks bring everything together in a single surroundings. Hereโs how it works:
- Data integration: You can integrate records from unique facts resources consisting of databases, data lakes, and cloud garages. This makes it clean to accumulate all of the data in a single location.
- Processing: Once facts are in Databricks, you can clean and edit them using easy indexing or integration equipment. This step facilitates making certain that your data is prepared for evaluation.
- Analysis and Visualization: After processing the data. You can analyze and create visual reports to understand trends and patterns. Databricks has tools that make this easy.
Traditional ETL and Modern Approaches: Which One is the Better Choice?
Traditional ETL:
- Batch Processing: Traditional ETL tactics have a tendency to work in batches. This way those processes collect and method facts at set times (together with overnight), which may cause delays in receiving updates.
- Complex setup: Traditional ETL setup can be time-consuming and requires specialized capabilities. An enterprise may additionally need a dedicated group to manage this technique.
- Limited flexibility: as soon as established, traditional ETL workflows may be rigorous. This makes it tough to evolve to new records assets or changing enterprise necessities.
Modern ETL:
- Real-time processing: Modern methods often allow real-time data processing. This means that the data can be continuously updated. It provides more timely insights.
- User-friendly tools: Many modern ETL tools are easy to use even by non-technical people. This allows team members to be more involved in data processing.
- Flexibility and scalability: Modern ETL solutions can quickly adapt to new data sources and larger volumes of data. This makes it ideal for businesses that are rapidly growing or changing.
ETL workflows are the backbone of effective data management. Although traditional ETL techniques are steeply priced, cutting-edge strategies offer speed, flexibility, and ease of use. This allows groups to make better decisions with information.
Benefits of Databricks to Boost ETL Efficiency
Databricks optimizes ETL operations through key features. This is especially useful for organizations interested in optimizing Databricks for ETL work.
- Speed: Databricks uses powerful cloud computing. This means they can process large amounts of data faster than traditional systems. Help companies gain insights faster.
- Collaboration: Teams can work together in real time on the same project. This collaboration reduces misunderstandings and speeds up the ETL process because everyone is on the same page.
- Automation: Databricks allow users to automate repetitive tasks such as data loading and cleansing. This means less manual work and fewer errors. It makes the whole process smoother.
Databricks Key Features for Seamless Data Integration
Databricks offers many features that make data integration and transformation easier:
- User-friendly interface: The platform has a user-friendly interface. This means that users can quickly understand how to use it. Even if they are not technical experts.
- Multi-Language Support: Databricks supports a wide range of programming languages, including SQL, Python, and R. This flexibility allows teams to use the tools they are most comfortable with for data transformation.
- Built-In Libraries: It comes with many pre-built functions and libraries for data processing and analysis, which helps streamline the transformation steps. This saves time and effort when coding.
- Seamless Integration: Databricks can easily connect to different data sources, whether they are databases, data lakes, or cloud services. This makes it simple to gather and integrate data from various places.
Databricks vs. Traditional ETL: Revolutionizing Real-Time Data Processing
Databricks stands proud of its ability to control actual-time statistics processing in several methods:
- Stream Processing: This isnโt the same as conventional ETL gear that frequently requires batch processing. Databricks can method facts because they are available. This approach means that companies can reply immediately to new data.
- Delta Lake: Databricks uses the Delta Lake feature to make sure records are always updated and consistent. This allows for reliable actual-time statistics processing without the usual headaches.
- Scalability: As the commercial enterprise grows, Databricks can scale to aid greater statistics and users immediately. This makes it perfect for environments in which information must be continuously processed.
- Instant Analysis: Databricks allows customers to run instantaneous analytics and create insights with new record streams. This is a key gain over conventional gear that may require looking ahead to the kit to finish.
Databricks improves ETL workflows with speed improvements working together and automation of various features. It helps increase the efficiency of data integration and transformation. and supports real-time data processing. This makes it a powerful choice for modern businesses looking to get the most out of their data.
Success Stories of Companies Thriving with Databricks ETL
- Comcast: One of the largest telecommunications companies in the world employs Databricks to solve business intelligence from the myriad of customersโ data. The primary benefit Comcast can get out of the more efficient ETL processes is that they can spot trends in customer interactions on a much shorter timeline, making the organizationโs improvements in service and product offerings easier.
- Regeneron: One of its large biotechnology clients, Regeneron uses Databricks for operating and analyzing large datasets in their research. With Databricks, they can work faster, thus leaving more time for researchers to work on ideas and not on data handling.
- Shell: Royal Dutch Company Shell for and real-time analysis of data uses Databricks. Shell is able to operate and manage resources in a better and faster manner because it analyzes and synthesizes data from different sources and forms.
Unlocking ROI Through Databricks Transformation of ETL Processes for Businesses
- Faster Insights: Details such as client data handling have improved dramatically, where companies such as Comcast have managed to cut through their time to analyze data processing time. This speed has implications in problem solving where greater revenues are realized through enhanced customer satisfaction.
- Cost Savings: Through ETL automation, companies will not require so much manpower and towing to accomplish the processes. For instance, Regeneron has reduced the costs of data preparation to a minimum they can afford, leaving a lot of room for budgets in other fields such as research.
- Improved Data Quality: Processing of data is error-free when companies adopt Databricks. It has been argued many times that better data quality results in the better quality of insights and predictions, which improve profitability on the operational level. For instance, Shell improves data accuracy that assists in optimizing operations, thus achieving immense cost reductions.
- Scalability: Since the growth of business, the associated data grows as well. With Databricks, it becomes easier for companies to scale their ETL process seamlessly. This means that as the load of data increases, the companies will not require a change of system to accommodate the changes, thus getting value for their investments in Databricks.
Companies like Comcast, Regeneron, and Shell demonstrate how to use Databricks for ETL to get the maximum benefits of Databricks for ETL. As a result, these companies have realized a high ROI since they have gained answers faster, spent less, received better-quality data, and made certain it is scalable for todayโs big-data world.
Databricks vs Popular ETL Tools A Simple Overview
- Ease of Use: The platform is easy to use, namely, the user does not or cannot be an expert in data. While tools like Talend and Informatica have their strengths, the problem is that they are relatively complex to set up as well as to use. Their users find that Databricks simplifies their work and allows for collaboration within a team without prior training.
- Cloud-Native: Databricks natively runs on the cloud, meaning that it can make use of high computing power effortlessly. On the other hand, in spite of the cloud variants offered by both Talend and Informatica, the two are inherently designed for on-premise deployment, which can prove a disadvantage due to the rigidity of the architecture.
- Real-Time Processing: Databricks has exceptional use cases for real-time data, and its processing makes it possible to analyze data in real time. Another form of tool, such as Informatica, for instance, focusses more on batch processing, hence slowing down the flow of insights and decision-making.
- Integration with Data Science: With Databricks, data science and machine learning tools are very easily constructed. This means that businesses can not only capture, clean, and store data, but they can build models from that data as well, all in one place. This capability is not as developed in other established ETL tools, such as Talend.
Read More: Databricks vs Snowflake! Understand the key difference
Databricks vs. Traditional ETL Unique Advantages Revealed
- Speed and Performance: Databricks relies on Apache Spark, a technology that enables the small time for data processing. This means that even large datasets can also be processed in record time, thus enabling business organizations to achieve understanding much faster than with the conventional ETL solutions.
- Collaboration Features: Part of its features, Databricks features collaboration capabilities through which two or more team members can work on a project at one go. This minimizes blocking and makes work more collaborative, which is sometimes a problem with conventional ETL systems.
- Flexibility and Scalability: Databricks also does not impose a heavy burden of scaling up to accommodate increased amounts of data as the companyโs data storage capacity increases. Traditional ETL solutions that have had data loading problems may find it hard to handle growth or changing business environments.
- Integrated Data Lakehouse: The platform by Databricks that integrates the idea of data lakes and data warehouses is called a โlakehouseโ. Thus, users can bring together aggregate and disaggregate information, as well as perform analytics on them without data transfers.
As for the specific reason why Databricks remains quite different from other ETL tools such as Talend Informatica, it is quite clear: Databricks is easy to use, natively cloud, real-time processing at its best, and data science integration is seamless. These great benefits, such as speed, collaboration opportunities, and flexibility, make it a perfect solution for todayโs businesses to improve ETL.
Getting Started with Databricks ETL Simple Steps to Success
- Define Your Goals: Databricks is fundamentally an Azure platform for building data science applications, and to get started, you first need to define what you want to accomplish with it. Do you need automated data processing, enhanced analytics, or to train a machine? Here are a few things you need to do. Having clear goals will help guide your implementation.
- Set Up Your Databricks Account: Create a trial account with Databricks. You can have options in cloud providers where you can select among AWS, Azure, or Google Cloud depending on your organization.
- Gather Your Data Sources: Determine how and where your data is currently situated. Such may involve the use of databases, cloud storage, or even external APIs. In the ETL process, specifically in the extraction phase, it will be useful to know what sources of data are available.
- Create a Team: Collect a group of interconnected people from the technical field: data engineers, analysts, and scientists. It will also be an advantage in the sense that there will be a variety that will enable you to cover all the aspects of data processing and analysis.
- Start with Sample Data: Having decided on the data analysis tools, test them on sample data prior to starting working with your main datasets. By practicing on this data, your team will be familiar with the Databricks layout and utilities without the added stress of working on actual information.
- Plan Your ETL Workflow: Identify your ETL workflows to determine how you will extract, transform, as well as load data. This is needed to achieve efficiency and effectiveness, as witnessed with Databricks workflows.
Read More: Solving Data Lake Challenges with Databricks Delta Lake
Whatโs Next? Key Trends in ETL and Data Processing for Businesses
- Real-Time Data Processing: Numbers of businesses are moving towards real-time analytics. This means they want to be able to perform online analysis as opposed to your batch analysis, where data is analyzed at one particular interval. Instant information provides a better opportunity to make faster, right decisions for companies.
- Data Lakehouse Architecture: People are talking about data lakehouses. This is a combination of data lakes that allow storing big amounts of unprocessed data and data warehouses that offer large amounts of structured data after their processing. It can accept different types of data at once, and that is something that makes it very useful in businesses.
- Increased Use of AI and Machine Learning: It is emerging that companies are interested in embedding AI and machine learning in their ETL processes. This makes data cleansing, data transformation, and even predictive analysis to be done smarter and automatically.
- Cloud Adoption: Cloud is becoming increasingly popular for data operations in organizations. This makes it flexible, easily scalable, and cheap, unlike the traditional means of business growth that are limited by physical hardware.
- Data Privacy and Governance: Due to rising legal restrictions regarding data usage and protection, organizations have to think about secure processing and management of data. It is a realization that accountability for handling data is assuming crucial importance.
Databricks Empower Your ETL Success with Ksolves Expertise
Databricks makes it easier to transform your ETL workflow with Ksolves by your side. We have a dedicated team of experts who will provide you with Databricks Professional Services that meet your needs. We assist you in adopting and enhancing journaling for organizational advantage. If you are willing to diversify your computing experience and get relevant results, call Ksolves now. Let us unleash the power of your data.
AUTHOR
Big Data
Anil Kushwaha, Technology Head at Ksolves, is an expert in Big Data and AI/ML. With over 11 years at Ksolves, he has been pivotal in driving innovative, high-volume data solutions with technologies like Nifi, Cassandra, Spark, Hadoop, etc. Passionate about advancing tech, he ensures smooth data warehousing for client success through tailored, cutting-edge strategies.
Share with